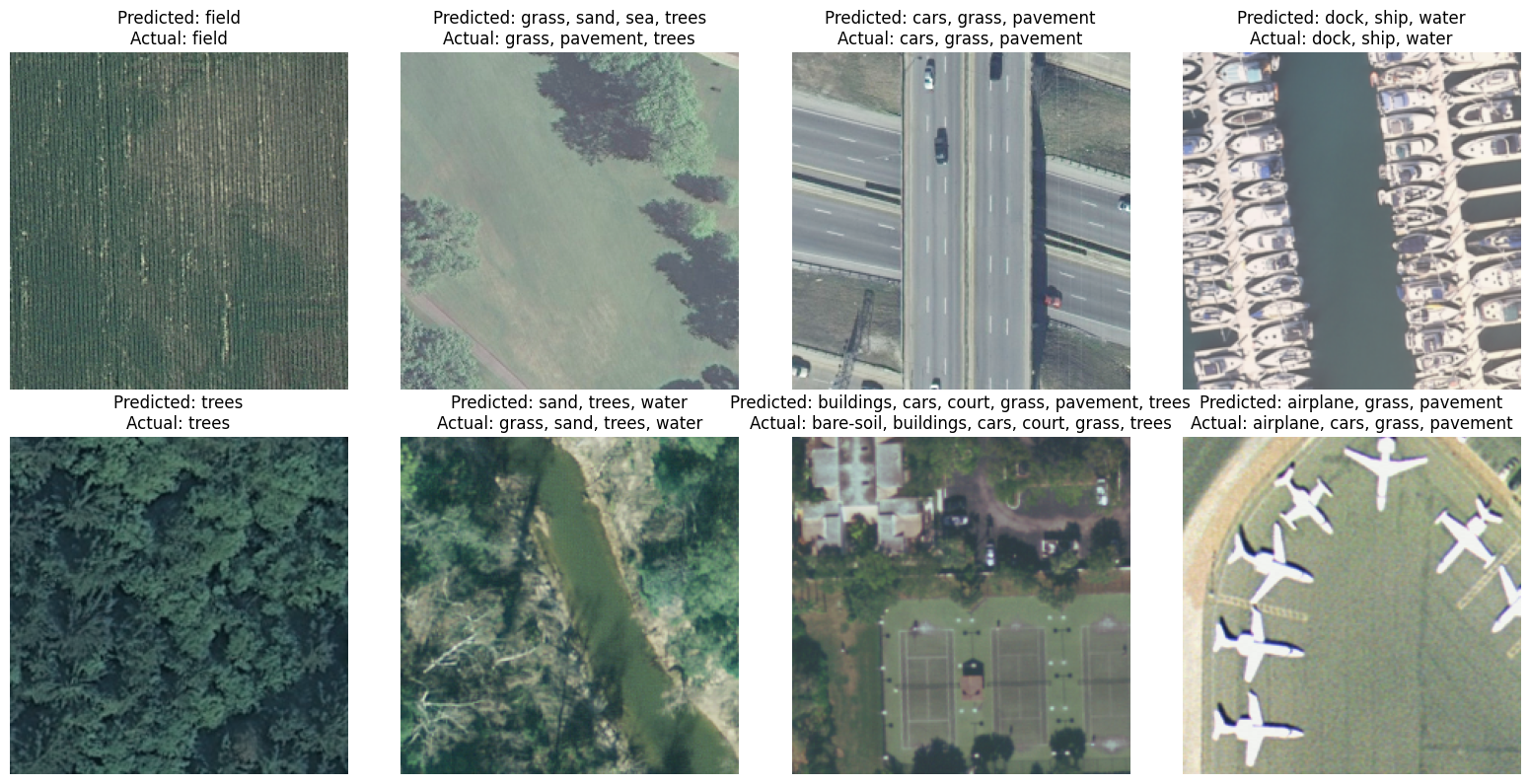
Prediction and true labels
Visual comparison between true labels and prediction.
In 2016, the Golden Jackal was first sighted in the Netherlands. It was necessary to know how the suitable habitat areas are linked to ensure genetic diversity of the species without having any conflict with wolf for habitat space and any obstacle for their paths. GIS tools identified movement paths and locations where the connections between suitable habitats are obstructed by water
It is beneficial to understand land class using satellite data. Machine learning algorithm enables us to map land classes.
In addition to machine learning, deep learning is also beneficial for land management. In this case, it was used to detect multiple objects in a single image. I applied several deep learning models, then achieved more than 90% f-1 score by the fine-tuning model of MobileNet3.
Visual comparison between true labels and prediction.
Thanks to use of pre-trained MobileNet3, high accuracy and low loss were achieved. Interestingly, validation results is always better than those of training.
Confusion matrix is a good tool to understand the performance of the model. In this case, the model is good at detecting 'tree' and 'building' but not good at 'road' and 'water'.
For single label classification task, Fine tuning of pre-trained ViT achieved 97% accuracy.
Biodiversity is the key to tackle climate change. It is important to understand the biodiversity of the area and educate people to protect it. Check out the concept of our Canopy Chase app to achieve this goal below (* only accessible from WUR students and teachers):
Canopy Chase Concept video
Canopy Chase App